Google DeepMind uses AI tools to discover 2.2 million new crystal structures, more than 45 times the number discovered so far
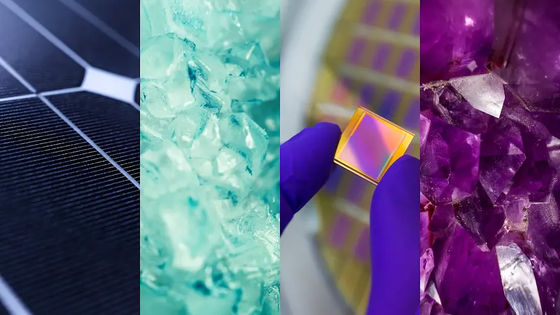
Google's AI research division,
Scaling deep learning for materials discovery | Nature
https://www.nature.com/articles/s41586-023-06735-9
Millions of new materials discovered with deep learning - Google DeepMind
https://deepmind.google/discover/blog/millions-of-new-materials-discovered-with-deep-learning/
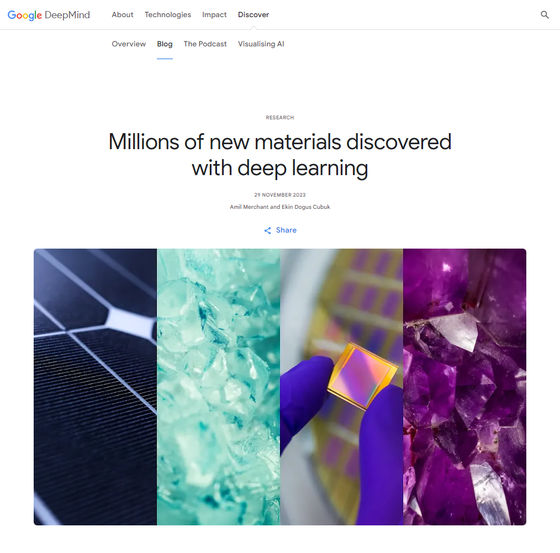
Google's DeepMind finds 2.2M crystal structures in materials science win | Ars Technica
https://arstechnica.com/ai/2023/11/googles-deepmind-finds-2-2m-crystal-structures-in-materials-science-win/
Modern technology, from computer chips to batteries to solar panels, relies on inorganic crystals. This is because in order to realize new technologies, crystals must be stable, otherwise they may break down. Obtaining new, stable crystals has required months of precise experimentation.
In a paper published in the scientific journal Nature on November 30, 2023 local time, the Google DeepMind research team shares that they have discovered 2.2 million new crystal structures. The discovery of new crystal structures uses Graph Networks for Materials Exploration (GNoME) , a deep learning tool that dramatically increases the speed and efficiency of discovering new materials by predicting their stability. .
There are 2.2 million new crystal structures discovered using GNoME, of which 380,000 are expected to be structurally stable. It seems that these crystal structures contain materials that have the potential to lead to innovative technologies such as superconductors, supercomputers, and next-generation batteries.
'For me, materials science is basically where abstract thinking meets the physical universe,' said co-author Eakin Degas-Kubek. 'Any technology can be improved if there are better materials. It will improve,” he said.
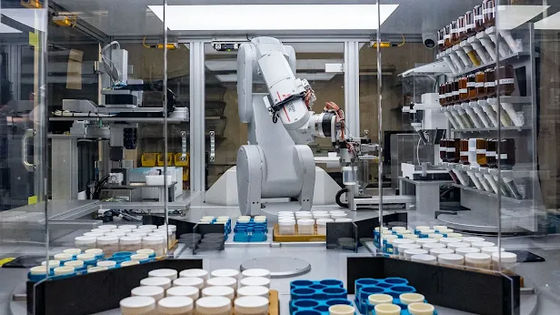
GNoME uses machine learning to identify new crystal structures by first generating candidate structures and then evaluating the stability of those structures. Google DeepMind notes that the number of stable substances discovered in the past 10 years is 28,000, so the discovery of new materials using GNoME is ``equivalent to 800 years of knowledge.'' did.
GNoME is a state-of-the-art graph neural network (GNN) model, and input data takes a graph format that can be compared to connections between atoms. Therefore, GNN is particularly suitable for discovering new crystal structures. GNoME is trained on data about crystal structures and their stability, and in order to evaluate the predictive power of the model during progressive training cycles, we understand the structure of the atoms, which is important for assessing stability. Iteratively checks the performance of crystal structures using an established computational technique known as density functional theory (DFT) used in physics, chemistry, and materials science.
Google DeepMind employed a training process called 'active learning' to dramatically improve GNoME's performance. GNoME generates new stable crystal structure prediction data, tests it using DFT, and uses the results as high-quality training data to train GNN models.
The discovery rate for material stability predictions measured using external benchmark tests is approximately 50% for state-of-the-art models, but GNoME has achieved a very high 80% discovery rate. Additionally, multiple laboratories have independently synthesized the new crystal structures discovered by GNoME, proving that the predictions are correct.
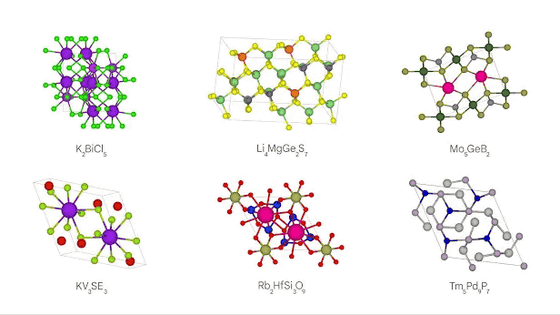
Related Posts:
in Science, Posted by logu_ii