What is the theory that supports the next generation of AI, claiming that 'deep learning is not all-purpose'?
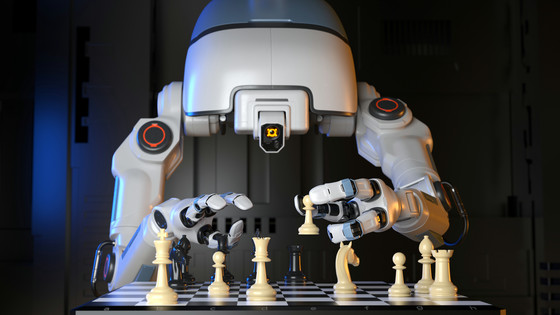
Advances in technology have led to the application of deep learning to commercial applications, further advancing the research and application of artificial intelligence (AI). However, while AI technology has made it possible to do things that were technically difficult until now, there are also cases where AI is overconfident and tries to solve anything with AI. The IEEE , an academic research organization in the field of electrical and information engineering in the United States, has published a column stating that 'deep learning is not all-purpose.'
Deep Learning Can't Be Trusted, Brain Modeling Pioneer Says --IEEE Spectrum
https://spectrum.ieee.org/deep-learning-cant-be-trusted
Typical deep learning programs do not perform well on multiple tasks and are well suited for performing specific tasks in a tightly restricted environment. In addition, deep learning is so complex that even if it works perfectly, 'I can't explain why it works perfectly,' IEEE said. Also, as you continue to study, some of what you have learned may suddenly collapse. Therefore, the IEEE argues that applying deep learning to life-threatening applications such as medical care may be risky.
Our brains learn to recognize and predict different objects and events in a changing world full of unexpected events. Therefore, there are many problems that cannot be solved by conventional deep learning that performs limited tasks in limited places.
One of the problems with classic AI, says IEEE Fellow Stephen Grossberg, is 'not trying to imitate the results of brain processing, but trying to find the mechanisms that produce the results.' I point out that. According to Grosberg, it is thanks to a special circuit in the brain that people can act immediately in response to new situations and sensations. People can learn from new situations, and unexpected events will be incorporated into the collected knowledge and prediction system for the world.
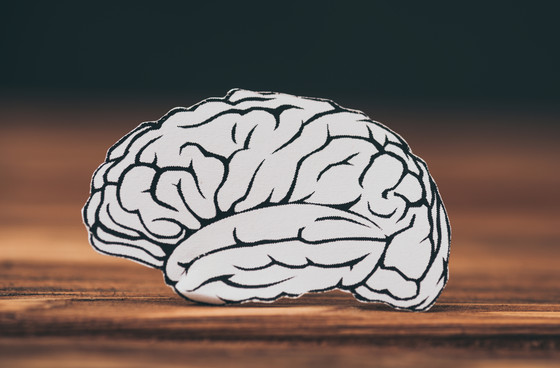
'The classic AI approach assumes that people can introspect the internal state of the brain using the concepts and words they use to express things and behaviors in their daily lives. It's a simple approach, but as a result, it was often inadequate to build a model of how the biological brain actually works. '
So Grosberg proposed the Adaptive Resonance Theory (ART) , an alternative model for both biological and artificial intelligence. In short, ART is a model of 'how the brain adapts to new situations and sensations and learns.'
'Understanding how the brain creates the mind is also important in designing smart systems in computer science, engineering, and high-tech fields such as AI and smart robots. ART helps us understand the brain. Not only can it be applied to the design of intelligent systems that can autonomously adapt to a changing world. ' In fact, ART solves problems such as pattern recognition and prediction with supervised and unsupervised learning. ART is actually applied to sonar and radar signal classification, sleep apnea syndrome detection, movie reviews, and driving support software by video analysis.
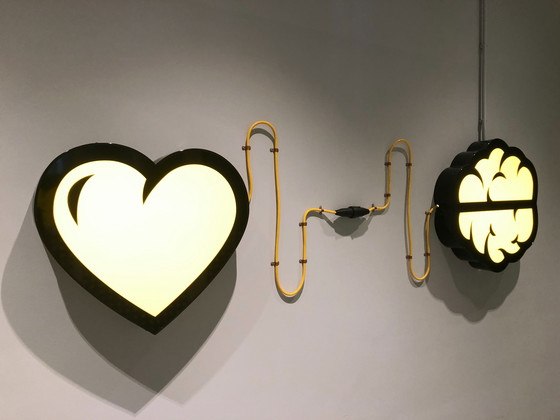
'The future of technology and AI will become increasingly dependent on such self-control systems,' Grossberg said. 'Self-control systems like AI are already in place, such as in the design of autonomous vehicles and planes. Deeper insights into brain design, heavily funded industry research and applications. I'm excited to think about how much can be achieved if it is incorporated into. '
Related Posts:
in Software, Posted by log1i_yk