'Why people dream' can be explained from the perspective of machine learning
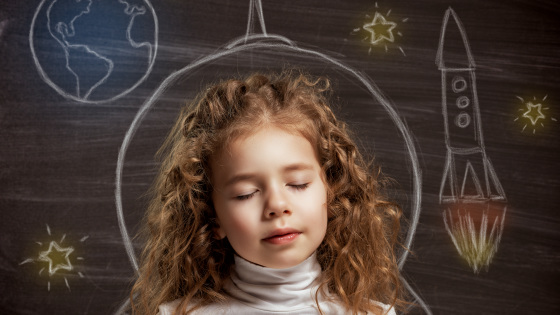
Everyone sometimes has ridiculous 'dreams' that are impossible in reality, but the reason for 'why do you dream' is not clear, and various hypotheses have been made.
The overfitted brain: Dreams evolved to assist generalization: Patterns
https://www.cell.com/patterns/fulltext/S2666-3899(21)00064-7
Our Weird Dreams May Help Us Make Sense of Reality, AI-Inspired Theory Suggests
https://www.sciencealert.com/ai-research-suggests-weird-dreams-might-help-our-brains-keep-reality-in-check
In machine learning, it is expected that learners such as computers will be able to perform some tasks based on the results of learning and training based on a large amount of data sets. However, if the learning period is too long or the training data is not appropriate, the learner will fall into a state of ' overfitting' in which he / she learns features that are unrelated to the data that should be learned.
When overfitting occurs, the learner cannot generalize the learning model and cannot output predictive data accurately. To eliminate overfitting, you can think of methods such as 'adding a data set', 'correcting the data such as inverting it if it is image data', and 'simplifying the data', but the most common method is Hoel says the traditional means is a 'dropout' that ignores certain data.
A common dropout technique is to randomly ignore some of the nodes in the dataset during training, which prevents the ignored data from affecting the predicted data and the model so far. It is said that it may also help to fit data that has never been seen in.
Hoel applied this to dreams and proposed the 'overfitting brain hypothesis' that machine learning and DNN overfitting are one of the reasons animals dream. In other words, animals dream because, in addition to the existing hypothesis that 'occurs to predict real-life events', 'occurs to prevent overfitting of the brain'. ..
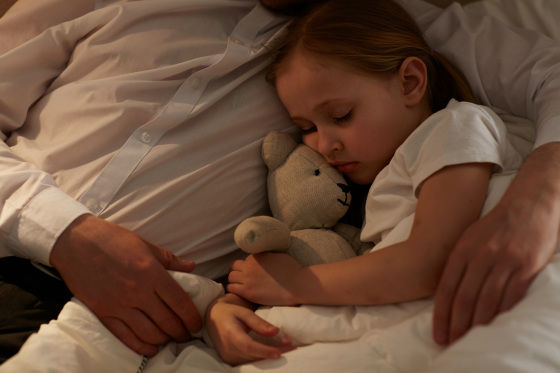
Dreams sometimes become unrealistic because the brain does not reflect all the events that it remembers while awake, but omits some detailed information. And that. Hoel explains that the lack of detailed information in dreams generalizes learning models, similar to machine learning, and simplifies the information processing that the brain does.
'The properties of dreams and machine learning have surprising similarities, and the overfitting brain hypothesis can be useful in both the fields of neuroscience and deep learning,' Hoel said.
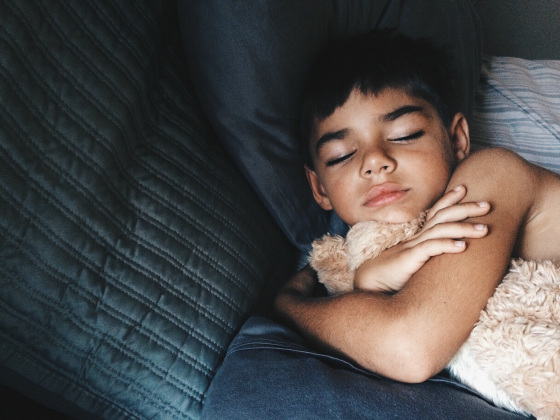
Related Posts:
in Science, Posted by log1p_kr