Google explains how to apply satellite images to solar radiation assessment to achieve efficient solar power generation
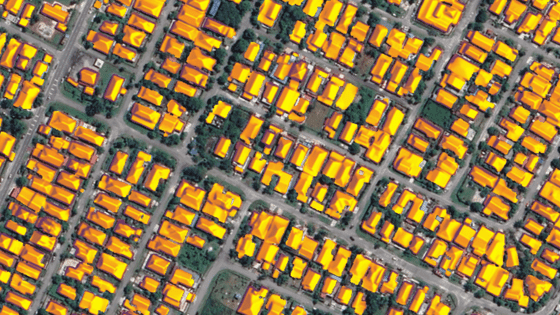
Energy demand has been increasing rapidly in recent years, and
[2408.14400] Satellite Sunroof: High-res Digital Surface Models and Roof Segmentation for Global Solar Mapping
https://arxiv.org/abs/2408.14400
Satellite powered estimation of global solar potential
https://research.google/blog/satellite-powered-estimation-of-global-solar-potential/
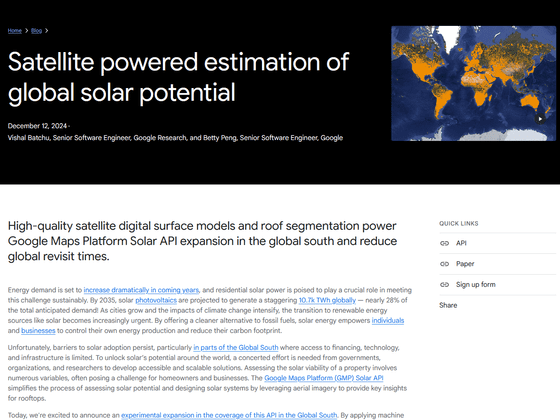
Installing residential solar panels can be time-consuming and complicated, as it requires educating homeowners, manually measuring and designing the solar panel installation, among other tasks, especially in emerging markets where data is limited.
Therefore, Google will release the 'Solar API' in 2023, which builds solar data based on images of building rooftops taken from an aircraft. This makes it possible to optimize designs using 3D models, realize efficient solar panel layouts, and reduce costs.
Until now, the Solar API was only available in Japan, the United States, and Europe, but due to the growing need for solar power generation in emerging markets, Google is investigating the application of satellite-captured images to the Solar API. Satellite-captured images have a lower resolution than aerial photographs, and have various problems such as a lack of accurate elevation maps, poor image quality, and distortion due to being taken at an angle. On the other hand, applying machine learning models to satellite images makes it possible to generate digital surface models (DSMs) and roof segmentation maps, expanding the scope of solar radiation assessment on a global scale.
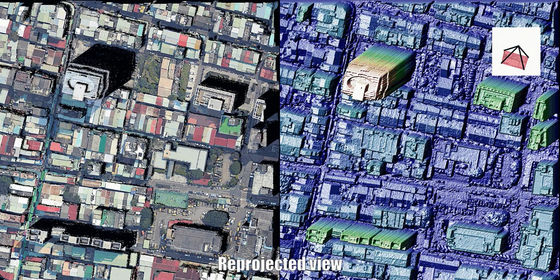
To apply the Solar API to satellite imagery, Google has developed a new machine learning model that generates high-quality height maps and roof flattening instances from a single image. It has also developed a model that makes it easier to visually understand height based on the distortion of the captured image.
These models are quantitatively evaluated using various metrics such as
By using satellite images, Solar API is able to evaluate the sunshine in various countries. Below is a graph showing the error of the height map by country. According to Google, the variation between countries is small, but Chile and the Philippines have large errors due to noise issues in the ground truth data. Google says, 'This suggests that DSM can handle a variety of building styles and sizes, and complex roof structures that vary by culture.'
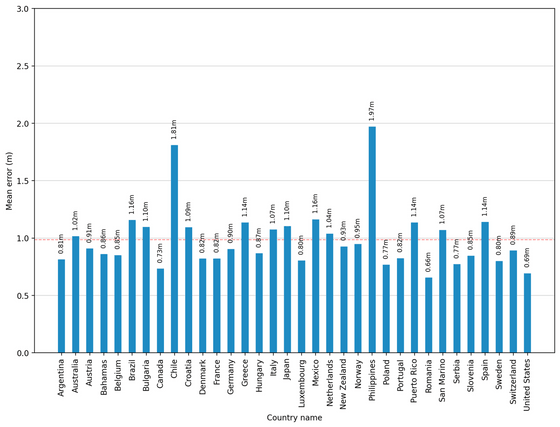
Google said, 'Our model generalizes well to a variety of architectural styles and landscapes. In areas with flat roofs, DSM accurately captures obstacles and roof surfaces with high accuracy, and in areas with many sloped roofs, DSM can effectively predict roof slopes.' Below are images taken by a satellite in Ayodhya, India (left), the image output by DSM (middle), and the final roof segmentation map image (right).
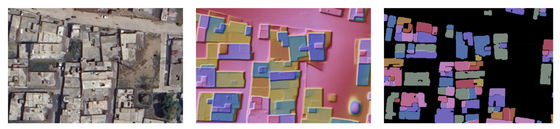
Below are images from Kuala Lumpur, Malaysia.
Here's a picture from Adelaide, Australia:
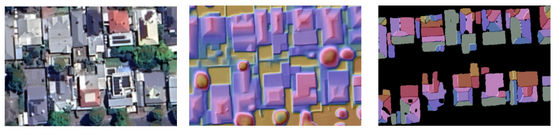
Google said, 'This expansion of the Solar API to satellite imagery significantly increases the availability of solar data, but factors such as input image resolution and cloud cover can affect the quality of the final output image. We are committed to improving accuracy through ongoing research and user feedback. Future research will also focus on exploring new research directions, such as detecting obstacles, detecting roof materials, and identifying existing solar panels.'
Related Posts:
in Software, Posted by log1r_ut