AI that can tell if a goat is in pain by looking at its face
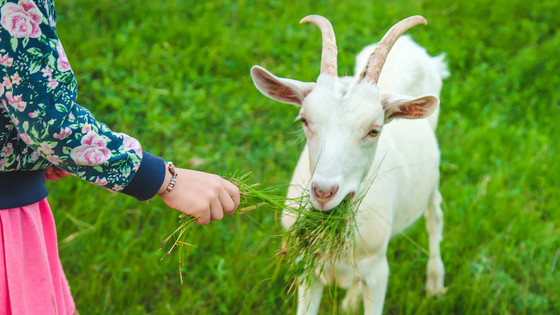
A research team from the University of Florida announced that they have developed a method to detect pain in goats using AI. They have succeeded in analyzing the facial expressions of goats in various states, from goats suffering from kidney stones to goats after surgery, and determining whether they are in pain or not.
Automated acute pain prediction in domestic goats using deep learning-based models on video-recordings | Scientific Reports
https://www.nature.com/articles/s41598-024-78494-0
Scientists train AI to detect pain—in goats
https://phys.org/news/2024-11-scientists-ai-pain-goats.html
To date, facial expression-based pain scales have been developed for a variety of animals, including mice, rats, cows, sheep, pigs, rabbits, and ferrets, and facial expression classification systems similar to the human Facial Action Coding System (FACS) have been developed for orangutans, chimpanzees, horses, dogs, and cats.
However, these assessment systems required manual annotation, which was time-consuming and expensive. They also required extensive training and certification of assessors, leaving room for subjective judgment. In particular, for goats, only pain scales that were limited to castration surgery for young, healthy males were available at the time of the study's start, and an assessment system that could be used in a wider range of situations was needed.
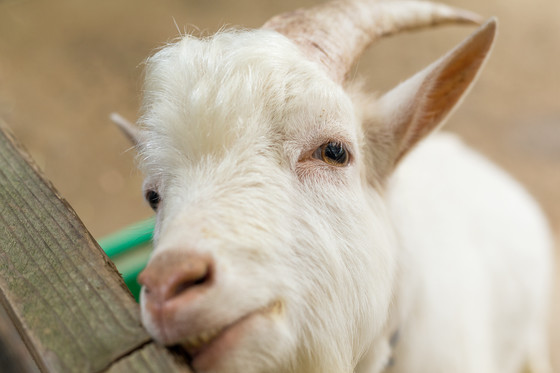
The research team collected video footage of 20 goats experiencing pain and 20 goats not experiencing pain, and used images extracted from the footage to train the model. Pain was assessed using the UNESP-Botucatu Goat Acute Pain Scale (UGASP), with a score of 3 or higher classified as 'painful.' The goats included were of various breeds, ages, and sexes, and the causes of pain included bladder stones and post-surgery.
In this study, we developed a model that combines a support vector machine classifier with a pre-trained convolutional neural network called VGG-16. Image extraction from video was tested in two patterns: 1 frame per second and 3 frames per second. As a result, although the accuracy varied depending on the validation method, single holdout validation and 5- fold cross validation achieved an accuracy of about 80%, and even the more rigorous individual 10-fold cross validation showed an accuracy of over 60%.
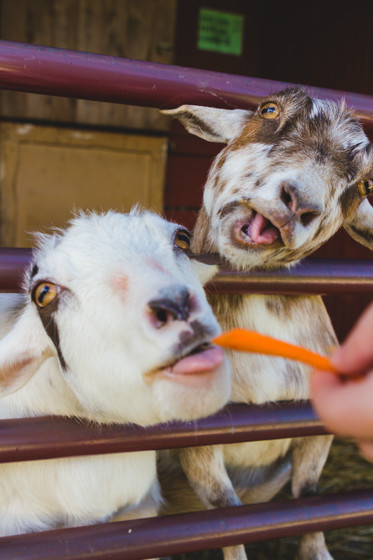
This research not only contributes to the development of an objective method for assessing pain in animals, but may also be applicable in the future to assessing pain in children and non-verbal patients who have difficulty communicating verbally.
In the agricultural sector, proper management of pain in livestock is expected to lead to improved animal welfare and improved productivity. The research team aims to collect more data and conduct further research in order to develop a practical pain assessment system for veterinary medicine.
Related Posts: