Researchers demonstrate 'CRAM' that reduces AI power consumption by up to 2500 times
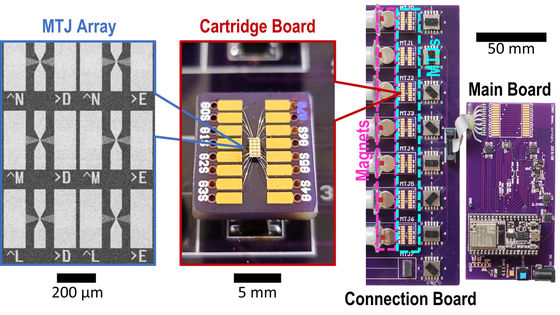
Researchers at the University of Minnesota, Twin Cities have demonstrated a hardware device that performs calculations directly inside memory cells, eliminating data transfers and delivering high performance at lower costs, potentially reducing AI energy consumption by up to 2,500 times.
Experimental demonstration of magnetic tunnel magnetic junction-based computational random-access memory | npj Unconventional Computing
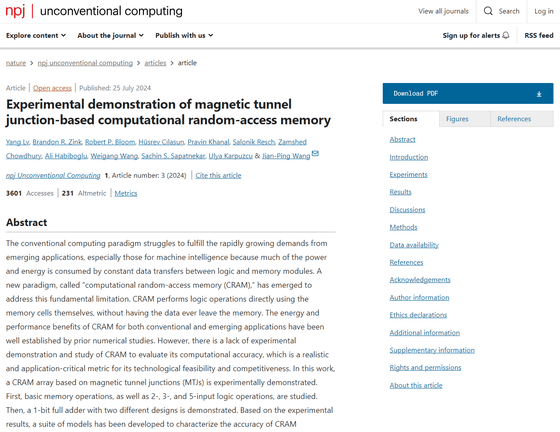
Researchers develop state-of-the-art device to make | Newswise
'Crazy idea' memory device could slash AI energy consumption by up to 2,500 times | Live Science
https://www.livescience.com/technology/computing/crazy-idea-memory-device-could-slash-ai-energy-consumption-by-up-to-2-500-times
In general 'computing', data is constantly moved between the processor that processes the data and the memory that stores the data. In the case of AI computing, the calculations are complex and large amounts of data are exchanged, so energy consumption is particularly high.
According to an annual report by the International Energy Agency (IEA), energy consumption by data centers, including AI, is expected to double from 460 TWh in 2022 to 1,000 TWh by 2026. This figure is equivalent to the total electricity consumption in Japan.
The International Energy Agency predicts that the electricity consumed by data centers around the world will rival that of Japan by 2026, and that energy demand doubled due to AI and virtual currencies will be covered by 'clean' electricity such as nuclear power - GIGAZINE
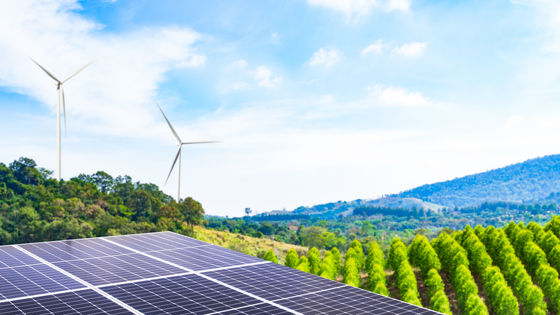
One thing that could improve this situation is 'CRAM (Computational Random Access Memory)' being studied by the University of Minnesota. The research has been going on for more than 20 years, and is funded by the Defense Advanced Research Projects Agency (DARPA), the National Institute of Standards and Technology (NIST), the National Science Foundation (NSF), and network equipment manufacturer Cisco.
'Twenty years ago, the concept of using memory cells directly for computing seemed crazy,' said Professor Jiang-Ping Wang , lead author of the paper. 'Since 2003, thanks to a growing group of students and a research team established at the University of Minnesota that spans physics, materials science and engineering, computer science and engineering, modeling and benchmarking, and hardware creation, we have seen encouraging results. We now demonstrate that this kind of technology is feasible and ready to be integrated into technology.'
CRAM is part of a longer-term effort based on magnetic tunnel junctions (MTJs), which Wang and his colleagues have worked on and patented. CRAM uses electron spin to store data, rather than relying on electric charge as in conventional memory, making it faster and more energy-efficient than conventional memory chips.
In addition, since it can handle a variety of AI algorithms, it is believed to be a solution that makes AI computing more flexible and energy efficient. In fact, when performing major AI tasks such as scalar addition and matrix multiplication, it was able to execute in 434 nanoseconds using 0.47 μJ of energy. This is about 1/2500 of the energy reduction compared to conventional memory systems.
Related Posts:
in Hardware, Posted by logc_nt