An attempt to accurately simulate a patient's blood circulation by using a 'digital twin' that reproduces a human on a PC is useful for a doctor's examination
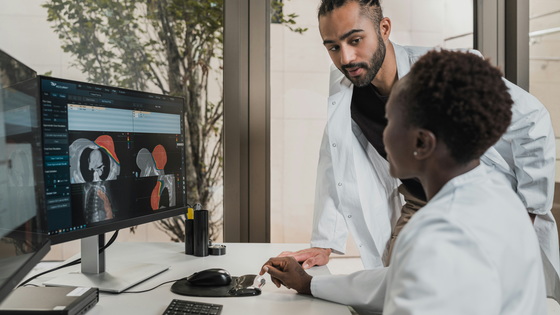
With 'Digital Twins,' The Doctor Will See You Now | Quanta Magazine
https://www.quantamagazine.org/with-digital-twins-the-doctor-will-see-you-now-20240726/
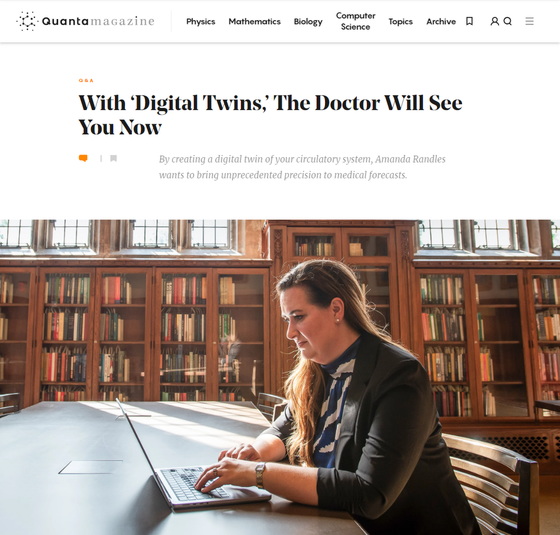
As a teenager in the 1990s, Randles was already envisioning a career that combined coding and biology. Randles then earned a degree in physics and computer science at Duke University, and after graduating, he worked at IBM for three years, developing the company's supercomputer, Blue Gene . After leaving IBM, Randles earned a PhD in applied physics at Harvard University, where he built a blood circulation model called 'Harvey' while in school. After graduating from Harvard, Randles got a job at Duke University and founded a laboratory to research the application of digital twins to medicine. In April 2024, Randles won the Computing Award from the Association for Computing Machinery , an international academic society in the field of computer science, for his research results, and received a prize of $250,000 (approximately 39 million yen).
Randles' blood circulation model takes 3D images of a patient's blood vessels and simulates the expected fluid dynamics. Doctors who use this blood circulation model will be able to monitor not only the usual measurements such as the patient's pulse and blood pressure, but also the predicted behavior of blood in the vessels. By using the blood circulation model, doctors can predict the 'eddies in the blood flow' and 'stress felt by the blood vessel walls' that are associated with heart disease. Until 10 years ago, Randles' blood circulation model could only simulate the blood flow of about 30 heartbeats, but as of 2024, it is able to simulate more than 700,000 heartbeats (about one week's worth). The blood circulation model can also simulate the blood flow of a patient who has taken prescribed medication, or the blood flow when a stent is added to improve blood flow.
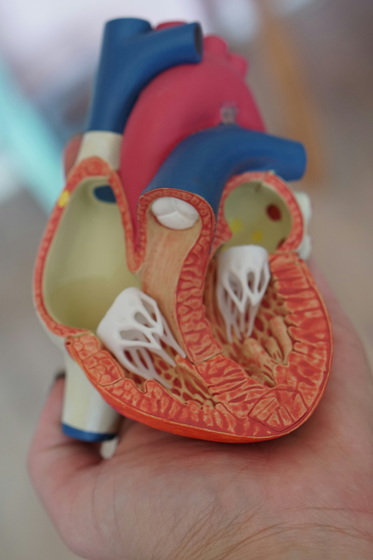
According to Randles, he first became aware of the potential of the blood circulation model when he published
A study of 160 patients demonstrated that Harvey could accurately predict patient measurements. The standard diagnostic method involves inserting a guidewire directly into the artery to measure the pressure before and after the stenosis to calculate the 'fractional flow reserve.' However, Randles said Harvey can measure this value non-invasively and accurately.
A major problem with the blood circulation model developed by Randles and his team is the sheer volume of data. Harvey simulates up to 580 million red blood cells, so the data size per model is likely to be as much as 0.5 terabytes (500 GB). In addition, a huge amount of calculation is required for each heartbeat, so arranging the computing resources is also a challenge.
Therefore, Randles and his research team are also researching methods to reduce the computing load using machine learning. The research team has developed technology that can train a model for simulating blood flow for each patient in a short time, and the technology can complete training in just 10 minutes, whereas existing FDA-approved tools take 24 hours to train. Therefore, it is possible to create a model in real time when a patient visits the hospital and use it for consultation.
To reduce training time, the team combined machine learning with smaller, physics-based models to calculate how many surgical treatment options need to be simulated for each patient to provide real-time predictions. Instead of using 3D models to train the machine learning, they used one-dimensional models, which reduced the amount of data required and shortened the training time.
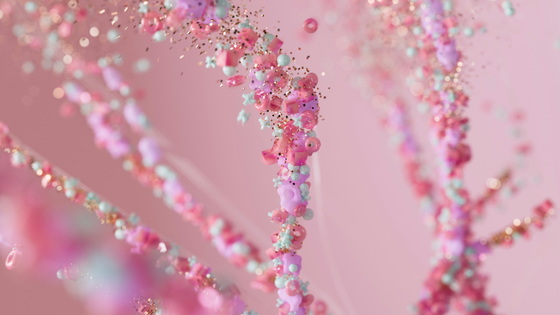
When asked about the limitations of applying digital twins to healthcare, Randles said, 'I don't think there are necessarily limitations, there are just a lot of challenges. There are a lot of things to tie together, like the nervous system and the lymphatic system. You want to integrate data from different systems, and getting them to talk to each other in a feedback loop is very complicated. But I don't think we can't get there.'
Related Posts:
in Software, Posted by logu_ii