Possibility of innovation in AI with the advent of ``neural network with human-like ability to generalize language''
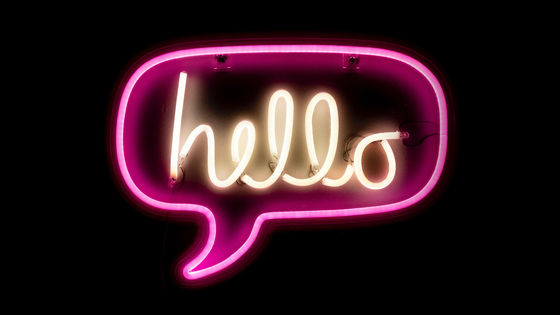
The success of developing a neural network that can perform '
AI 'breakthrough': neural net has human-like ability to generalize language
https://www.nature.com/articles/d41586-023-03272-3
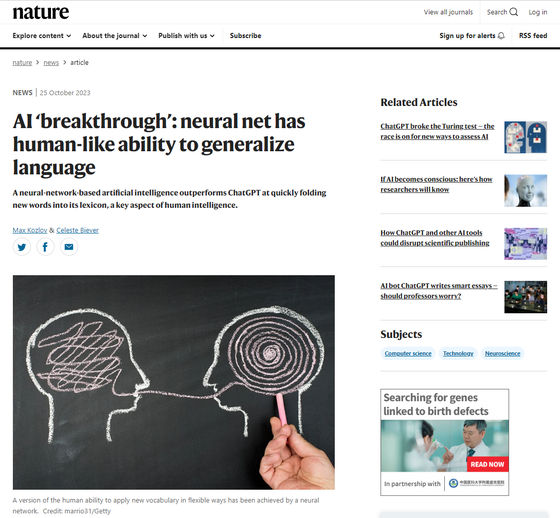
Chatbots shouldn't use emojis
https://www.nature.com/articles/d41586-023-00758-y
Humans interact with language by incorporating newly learned words into their existing vocabulary and using them in new contexts, a task known as generalization. Generalization is demonstrated by people's ability to use newly acquired words effortlessly in new environments. For example, if you understand the meaning of the word ' photobombing ' (the act of appearing in a photo as a prank), 'photobombing' can be used in various situations, such as 'photobombing twice' or 'being photobombed during a Zoom call.' You will be able to use the word. Similarly, someone who can understand the sentence ``The cat chases the dog'' should be able to understand the sentence ``The dog chases the cat'' without thinking about it.
But this ability to generalize in language is not innate to neural networks, the method of 'emulating human cognition' that has dominated AI research, says a cognitive computational scientist at New York University. This was revealed in a study by Brendan Lake et al. Unlike humans, neural networks have difficulty generalizing until they have learned multiple sample texts that use a word.
Lake and his team tested 25 participants to see how well they applied newly learned words in various situations. The research team tested the subjects' generalization ability by using a pseudolanguage consisting of two categories of meaningless words.
In the test, primitive words such as 'dax,' 'wif,' and 'lug' were set to represent basic actions such as skip and jump. We then set up words such as 'blicket,' 'kiki,' and 'fep' as more abstract functional words. By combining primitive words and functional words, we are able to create expressions such as ``jump three times'' and ``skip backwards.''
Next, subjects are trained to associate primitive words with circles of a certain color. Specifically, 'dax' represents a red circle and 'lug' represents a blue circle. The participants were then asked to answer what kind of circle they could make by combining the primitive words and the functional words. For example, we measured the ability to generalize words from two categories, such as ``dax fep'' with ``3 red circles'' and ``lug fep'' with ``3 blue circles.''
Testing has shown that human subjects perform better on language generalization tasks. It seems that the subjects succeeded in answering the combination of words representing the correct number of colored circles at an average rate of 80%.
The researchers then trained the neural network to perform tasks similar to those tested on human subjects. Rather than using static datasets, which is the standard approach for training neural networks, we allow the AI to train as it completes each task. To make neural networks more human-like, the research team is training them to reproduce patterns of error observed in human test results. After that, when they created a new test using the same rules and had the trained neural network perform the test, the correct answer rate was almost the same as that of humans, and in some cases it exceeded human performance.
On the other hand, GPT-4, on which ChatGPT is based, struggled in similar tests. Despite ChatGPT being able to have fluent conversations like humans, the average error rate was 42-86%, lower than humans or the aforementioned neural networks.
'It's not magic; it's learned through practice. Just like a child practices when learning their native language, the AI model improves its compositional skills through a series of compositional learning tasks,' Lake said. mentioned. In addition, Paul Smolensky, a cognitive scientist specializing in language at Johns Hopkins University, commented on the human-like performance exhibited by neural networks, saying, ``This represents a breakthrough in our ability to systematically train networks.'' It suggests that.'
Melanie Mitchell, a cognitive scientist at the Santa Fe Institute in New Mexico, said: 'While this study is an interesting proof of principle, it remains to be seen whether the training method can be generalized across larger data sets. No,” he said. On the other hand, Lake said, ``By researching how humans acquire the knack for systematic generalization from an early age and incorporating those findings to build more robust neural networks, we can solve this problem.'' I would like to work on this,' he said.
Elia Bruni, a natural language processing expert at the University of Osnabrück in Germany, said this research could improve the learning efficiency of neural networks. It also said that the huge amount of data needed to train things like ChatGPT will be reduced, minimizing the 'hallucinations' that occur when AI recognizes patterns that don't exist and creates inaccurate output. Ta.
Related Posts:
in Science, Posted by logu_ii