An AI learning method that can win 99% against the strongest Go AI ``KataGo'' opponent is devised, it is too special to be effective only for AI opponents
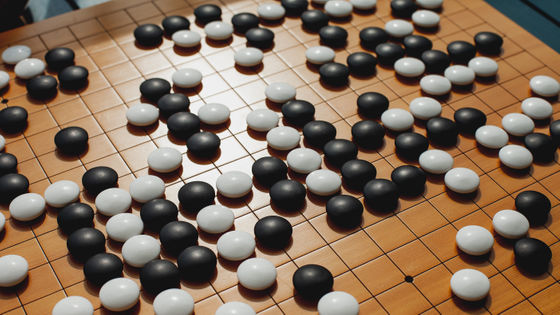
The cutting-edge Go AI '
[2211.00241] Adversarial Policies Beat Professional-Level Go AIs
https://doi.org/10.48550/arXiv.2211.00241
Adversarial Policies in Go - Game Viewer
https://goattack.alignmentfund.org/
Use magic strike magic! One shouting board level human chess hand chess AI has been transferred
https://it.sohu.com/a/602280072_129720
Starting with Go AI such as KataGo, recent competitive AI such as Othello and chess basically perform reinforcement learning by “self-play”. Self-play means to learn by “self” becoming an opponent. The self-play learning method is to come up with the most efficient way to move your own hand and your opponent's hand, and to always make the best choice no matter what kind of hand your opponent challenges. This is based on the concept of Nash Equilibrium , which states that if both players always make the most rational choices, they will not give up on each other's strategy.
This time, Mr. Wang and others prepared two KataGo that were trained by different methods and conducted an experiment in which they fought each other in Go. In the experiment, KataGo that learns by the same self-play as before is named 'victim', and KataGo that learns by a new method is named 'attacker'.
The new method devised by Mr. Wang et al. is to `` mutually analyze your own neural network on your turn and the opponent's neural network on your opponent's turn ''. Normally, in self-play, only 'your own neural network' is analyzed, but in the new method, each other's neural network is analyzed as shown on the left. By using such techniques, it was hoped that attackers would be able to discover the fundamental weaknesses of their opponents.
The results of the actual competition are as follows. In the game record below, white is the victim's stone and black is the attacker's stone. The strategy devised by the attacker is to ``put a small number of stones on the corners of the Go board to form a `` ground '' and dare to place one's own stones on the victim's ground.'' Under normal circumstances, it should be easy for the victim to take all the opponent's stones on his land and win a decisive victory, but the victim passes before finishing securing the ground. , I gave up. With this strategy, the attacker achieved a winning rate of 99% or more in the match against the victim.
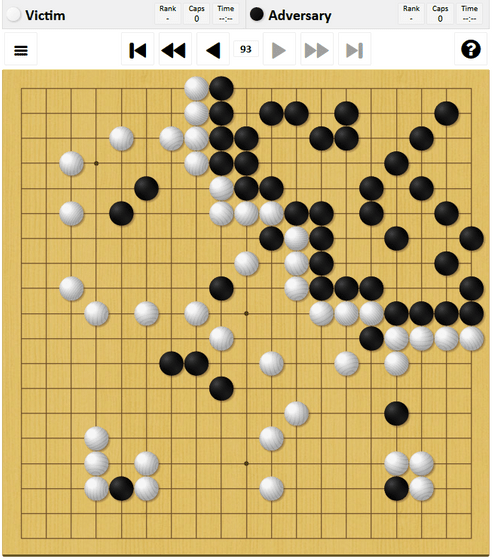
However, when the number of games reached 64, the winning rate dropped to 80%, and when it reached 8192, the winning rate dropped to 48%. Around this time, the attacker puts too many stones on the victim's ground, and the victim who has learned does not concede, so the game ends when the board is filled up. The white below is the attacker and the black is the victim.
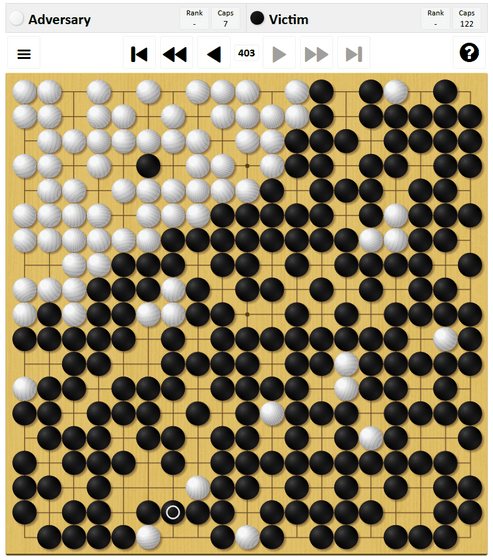
This strategy is only applicable to AI that performs self-play, and if the opponent is a human, even a beginner in Go can easily lose by a large margin. When Mr. Wang, who is actually a Go beginner, tried playing against this strategy, he won with a difference of more than 250 points. In the following game record, white is Mr. Wang and black is the attacker.
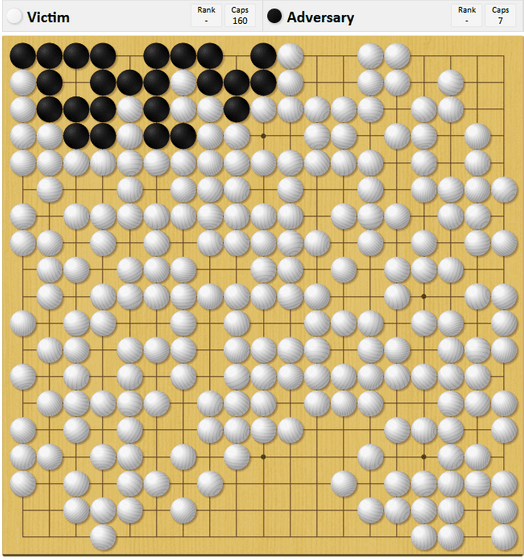
Based on the above results, Mr. Wang explains, ``The new strategy is strong against professionally learned AI, but cannot beat human players.''
In addition, Mr. Wang says that by taking a similar strategy, he was able to win the Go bot 'NeuralZ06', which reigns in the top 50 of the online Go site 'KGS'. However, if the `` friendlyPassOk function '' that `` players can pass without taking all the stones they can take '' was disabled, they could not win.
Wang et al. ``This result suggests that even highly capable AI may have serious vulnerabilities. Such findings in Go AI are interesting, but A similar vulnerability in a security-critical system like automated financial transactions or self-driving cars could have disastrous consequences. In order to create a model with high reliability, we should make every effort to develop a system that can compete with this kind of method.”
Related Posts: